JITE v46n3 - Motivation While Designing in Engineering and Technology Education Impacted by Academic Preparation
Motivation Vhile Designing in Engineering and Technology Education Impacted by Academic Preparation
Nathan Mentzer
Purdue University
Kurt Becker
Utah State University
Abstract
The purpose of this study was to determine if high school students’ academic preparation was correlated with change in motivation during an engineering design challenge. The research was conducted in a high school classroom in which elements of engineering design were taught in a technology education context to eleventh-grade student from diverse academic backgrounds (measured by grade point average [GPA]). Participant motivation was assessed by the California Measure of Mental Motivation (CM3). The CM3 measures student motivation to apply critical thinking skills and reasoning to solve problems in five subscales: mental focus, learning orientation, creative problem solving, cognitive integrity, and scholarly rigor.
Findings of this study suggested that knowledge of students’ GPA served as a predictor of student motivation. With the exception of the mental focus subscale, growth over time was not related to GPA. Change across multiple time points in the other four subscales of learning orientation, creative problems solving, cognitive integrity, and scholarly rigor did not show significant correlation with mathematics, science or communication GPA.
Introduction
High school technology education has developed a reputation for providing service to an academically diverse student body under the umbrella of general education. Technology education provides an opportunity to integrate academic material in real problem solving challenges ( International Technology Education Association, 2000 ). Students who excel in the academic areas of mathematics, science and communications find technology education a domain where their strengths are valued. Students who struggle through traditional academic material find context and relevance in technology education courses that may spur excitement and perseverance ( Lewis, 2004 ). In contrast, the study of engineering is intimately related to science and mathematics ( Katehi, Pearson, & Feder, 2009 ). High school students engaged in the study of engineering are often the most successful academically. These students tend to have been high achievers in the core academic content areas.
As the field of technology education considers integration and implementation of engineering (the central focus of which is design), attention must be given to the impact on all students. Will only highly motivated and successful students demonstrate gain in courses where engineering and technology intersect? What will happen to less academically prepared students who traditionally enroll (and succeed) in technology education courses? Will technology education effectively exclude a large subset of the student body by incorporating engineering design principles based challenging core academic areas?
Motivation and student learning have a dynamic and complex relationship. “The challenges of learning for today’s world require disciplined study and problem solving from the earliest grades. To meet the challenges, learners must be motivated to pay attention, complete assignments, and engage in thinking” ( Bransford, Brown, & Cocking, 2000 , p. 280). Students enter classrooms with a broad range of motivation. Student’s motivation has the potential to increase or decrease over time impacted by many factors including the learning experiences in class.
“Challenges, however, must be at the proper level of difficulty in order to be and to remain motivating: tasks that are too easy become boring; tasks that are too difficult cause frustration” ( Bransford, et al., 2000 , p. 61). Vygotsky suggested appropriate learning experiences fall within a student’s zone of proximal development (ZPD). This zone represents the difference between a student’s individual capability and the student’s potential with support from peers or teachers. Maintaining learning experiences in mixed courses of students challenges the educator to deliver developmentally appropriate materials for a wide range of student needs.
According to the STL ( Standards for Technological Literacy: Content for the Study of Technology ( International Technology Education Association, 2000 ), technological literacy is important for all students, and therefore technology education students represent a broad range of academic backgrounds. Thus, it is essential to understand how engineering design challenges affect all students from low to high achievers. If the pedagogical implementation of engineering design challenges is only successful for the highest achieving students, a disservice will be provided to students who are academically less prepared. Therefore, the following research question framed this inquiry: Does a student’s academic preparation correlate with the individual’s change in motivation during an engineering design challenge? If growth in student motivation is uncorrelated with an indicator of student success in school, infusing engineering concepts into technology education is likely to be successful for all students, which is consistent with the mission of ITEA as identified in Technology for All Americans ( International Technology Education Association, 1996 ).
Motivation
The authors reviewed nine studies that have been published in the past 15 years and focus on the efficacy of engineering design challenges. These studies were concerned with change in motivation among learners in elementary schools, secondary schools, and the college years ( Dally & Zhang, 1993 ; Dunlap, 2005 ; Griffith, 2005 ; Lentz & Boe, 2004 ; Ricks, 2006 ; Rogers, 2005 ; Romero, Slater, & DeCristofano, 2006 ; Roselli & Brophy, 2006 ; Weir, 2004 ). In these studies, motivation was assessed using a variety of instruments including course evaluations, surveys, instructor perceptions, and self-efficacy scales. Each study comparing a traditional teaching model to a teaching model employing elements of engineering design challenges showed improvement among students experiencing the engineering design approach. Four of the nine studies reported statistically significant gains (p < 0.05). Though improvement was demonstrated, these studies did not disaggregate the data by previous levels of academic performance in order to identify differential effects on more (or less) academically prepared learners.
The construct measured in this study was the motivation to apply critical thinking and reasoning skills to solve problems. The California Measure of Mental Motivation (CM3) was selected after consulting with the publisher, Insight Assessment. The CM3 measures a student’s motivation to apply critical thinking skills and reasoning to solve problems. Five areas were assessed, as explained by Insight Assessment:
- Mental Focus/Self-Regulation: The person scoring high in mental focus is diligent, focused, systematic, task-oriented, organized, and clear-headed.
- Learning Orientation: A person scoring high in learning orientation strives to learn for learning's sake; they value the learning process as a means to accomplish mastery over a task. These individuals are eager to engage in challenging activities. They value information and evidence gathering, recognize the importance of giving reasons to support a position, take an active interest and are engaged in school.
- Creative Problem Solving: The person scoring high in creative problem solving is intellectually curious, creative, has a preference for challenging and complicated activities, and is imaginative, ingenious, and artistic.
- Cognitive Integrity: Individuals scoring high in cognitive integrity are motivated to use their thinking skills. They are positively disposed toward truth seeking and open-mindedness.
- Scholarly Rigor: Scholarly rigor is the disposition to work hard to interpret and achieve a deeper understanding of complex or abstract material. A person with a high score on this scale exhibits a strong positive disposition toward scholarly rigor and would not be put off by the need to read a difficult text or to analyze complicated situations or problems. ( Insight Assessment, 2007b )
Validity and reliability of the CM3 instrument were considered during the instrument selection process. Reliability has been computed using the Cronbach’s alpha (refer to Table 1). In addition to reliability assessments, the CM3 has been studied for its external validity, predictive validity, and discriminant validity. Data supporting the validity is published in the User Manual ( Insight Assessment, 2007a , pp. 25-30).
Focus area | Cronbach’s alpha |
---|---|
Learning orientation | .79 - .83 |
Creative problem-solving | .70 - .77 |
Mental focus | .79 - .83 |
Cognitive integrity | .53 - .63 |
Scholarly rigor | NA |
( Insight Assessment, 2007a , p. 27) |
Research site
An eleventh grade high school course was identified which included an academically diverse array of students and provided a semester-long engineering design challenge. This team taught course was described in the syllabus:
This course will introduce many concepts of engineering and the designing of systems.… The labs will provide a bridge between what we learn in the classroom to practical applications in a real world setting. We will apply technology, and the skills we have learned in math, science and communication to several major projects.
This research setting provided student experiences in technology education focused on fabrication, as well as an understanding of the underlying science and math principles governing the physical world to enable them to design systems and components.
For purposes of this research, an engineering design challenge was defined as a team based activity in which students engage in solving a real world problem. In this engineering design challenge, mathematical models were developed to understand the behaviors of systems. The data extracted from manipulating models served to guide experimentation. Design decisions were made based on model and experimental results.
During the fall semester, teachers provided a foundational knowledge base for the spring term. Students participated in hands-on learning experiences which represent an intersection of technology education and applied physics. For example, students learned physics concepts such as motion, forces, electricity, magnetism and simple machines, as well as technology content such as welding, machining, mechanical fastening, and metal working processes. The concluding projects (1/10 scale model and mini-frame with jig) in the fall term set the stage for design and fabrication of a solution to the engineering design challenge that officially began with the spring term.
The spring term was initiated by assembling teams and focusing on defining the engineering design problem. The problem included the design and fabrication of an ultra efficient competitive electric race car following the Electrathon American design constraints (2007) . Teams of students started the engineering design challenge by refining their design based on the 1/10 th scale model of an electric car and driver. Then teams of 2 to 6 students designed, modeled, and built their full size Electrathon vehicle. Constraints were imposed by the Electrathon rule book and local facilities. Designs were optimized for minimal weight, tire scrub, air resistance, and other characteristics. Analysis was incorporated into the modeling in the form of model car wind tunnel testing, gear ratio calculation, power demand calculation, and ratios of battery life to distance traveled. Understanding of these parameters had been developed in the fall term by building and testing smaller projects such as magnetic levitation cars and calculating horsepower capacity of a student built electric motor.
Participants
The sample included 28 regular education students who completed the course. Female enrollment was 10.7% (n=3). Of the students who chose to report ethnicity, 75.0% (n=21) were Anglo American or Caucasian; 3.6% (n=1) Hispanic, Latino, or Mexican American, 3.6% (n=1) Native American and 17.9% (n=5) reported mixed or other. The proportion of students not reporting as Anglo American/Caucasian was 3.1% higher than the school statistic of 21.9% and comparable to the national demographic of 24.9% ( U.S. Census Bureau, 2000 ). Cumulative GPA had an overall mean of 2.24 on a scale of 0-4. Cumulative GPA ranged from 1.00 to 3.75 and had a standard deviation of 0.80. Participants serviced by special education accommodations were not considered in this analysis.
Methods
The research question for this study was to identify potential correlations between a student’s academic preparation and the individual’s change in motivation during an engineering design challenge. To address this research question, a repeated measures correlation study was conducted in which data were gathered on student motivation at three points during the academic year. Trends and changes during the year were compared to an indicator of each student’s academic preparation.
Data analysis was conducted using longitudinal multilevel modeling techniques. This analysis allowed multiple predictor variables to be analyzed in this repeated measures design for prediction of motivation. “…Applications of multilevel models are longitudinal research and growth curve research, where a series of several distinct observations are viewed as nested within individuals…” ( Hox, 2002 , p. 1). Predictor variables included high school grade point averages for core academic areas (science, mathematics, and communications), time, section, and demographic information.
In the modeling process, the main effects of predictors were considered in addition to their interactions with time. Interactions between main effects were analyzed including the effect of academic preparation and time. Slopes and intercepts of main effects and interactions were interpreted. This analytic modeling strategy facilitated an understanding of relationship between a student’s academic history and changes in motivation during an engineering design challenge.
A main effects only model was created and tested against a main effects model that included interactions of time and each predictor. Significance testing was conducted using likelihood ratio tests comparing the models using R. Modeling was conducted with R software version 2.7.0 and the linear mixed-effects models package version 0.99875-9 ( Bates, Maechler, & Dai, 2008 ). Significant interactions were included in a model which was then reduced in a top-down approach. A reduction technique was employed where the least significant predictors were removed one at a time. Each model iteration was compared to the previous model using likelihood ratio test to determine if it was statistically different. This process was employed for each of the five CM3 motivation subscales. First-level units were repeated measures within individual study participants. Data from 83 mental motivation tests were considered for analysis. Second-level units were 28 participants in this study. In the hypothesized models, individuals and time are declared random effects to assess variability among individuals within time points, as well as variability among time points.
Results
The CM3 measured five subscales of motivation: mental focus, learning orientation, creative problem solving, cognitive integrity, and scholarly rigor. Means for each subscale are presented in Table 2 and 4 of 5, show growth over time. Scales range from 0 to 50 and are interpreted by categorization as shown in Table 3. A two-level, longitudinal, multilevel model assessed the effects of grade point average in mathematics, science, and communication courses, course section, and minority status on mental motivation. It was expected that a potential correlation existed between change indicated by the CM3 and GPA.
October
n=28 |
December
n=27 |
April
n=28 |
||||
---|---|---|---|---|---|---|
M | SD | M | SD | M | SD | |
Mental focus | 28.50 | 8.23 | 28.07 | 8.27 | 28.50 | 8.27 |
Learning orientation | 32.43 | 5.88 | 32.30 | 7.75 | 33.96 | 7.92 |
Creative problem | 29.75 | 8.09 | 32.48 | 8.53 | 32.29 | 10.03 |
Cognitive integrity | 34.18 | 6.72 | 33.44 | 7.24 | 34.93 | 8.68 |
Scholarly rigor | 27.75 | 4.70 | 28.15 | 5.62 | 28.11 | 6.01 |
Average | 30.52 | 30.89 | 31.56 |
Score on CM3 scale | Interpretative category |
---|---|
0-9 | Strongly negative |
10-19 | Somewhat negative |
20-30 | Ambivalent |
31-40 | Somewhat disposed |
41-50 | Strongly disposed |
Note: Table adopted from California Measure of Mental Motivation Score Interpretation Document ( Insight Assessment, 2006 ) |
Mental focus
According to the CM3, a student scoring high in mental focus was diligent, focused, systematic, task-oriented, organized, and clear-headed. Mental focus scores did not significantly increase over time. A full model was developed which included main effects and significant interactions. A parsimonious fixed slope model was reduced from the full model which was not statistically different, χ 2 (3, N = 83) = 518.7– 516.7= 2.0, p > 0.05. No statistically significant main effects were present in the model.
A significant positive interaction was discovered between time and mathematics GPA. This suggested that students with higher mathematics GPA’s tended to gain more over time, illustrated in Figure 1, than did their peers with lower mathematics GPA’s. A significant negative interaction was discovered between time and science GPA, as shown in Figure 2. This negative interaction suggested that lower science GPA students tended to gain more over time than did their higher science GPA peers.
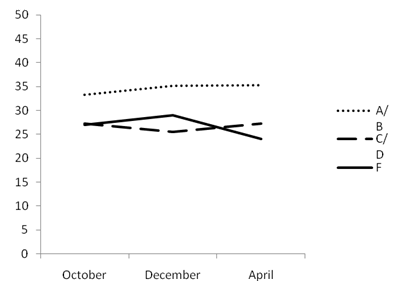
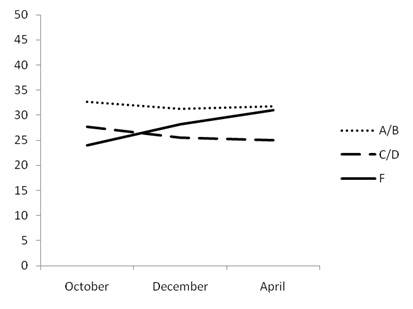
Learning orientation. A student scoring high in learning orientation was motivated by the desire to increase knowledge and skill base as published with the CM3. Learning orientation scores did not significantly change over time. A parsimonious random slope model was reduced from the main effects only model which was not statistically different, χ 2 (4, N = 83) = 530.1 – 531.5 = 1.4, p > 0.05. No statistically significant main effects were included in this model. No significant interactions were discovered with any predictor and time, which indicated no significant change over time related to student GPA.
Creative problem solving. According to the CM3, a student scoring high in creative problem solving had a tendency to approach problem solving with innovative or original ideas and solutions. Creative problem solving scores increased significantly over time. A parsimonious random slope model was reduced from the main effects only model which was not statistically different, χ 2 (3, N = 83) = 530.3– 527.2 = 3.1, p > 0.05. The statistically significant main effect in this model was time. Students’ GPA’s in science and communications improved the model fit significantly but were not statistically significant predictors. No significant interactions were discovered with any predictor and time, which indicated no significant change over time related to student GPA.
Cognitive integrity. A student scoring high in cognitive integrity was motivated to use thinking skills in a fair minded fashion, seek the truth, and be open minded. Cognitive integrity scores did not significantly change over time. A parsimonious fixed slope model was reduced from the main effects only model which was not statistically different, χ 2 (6, N = 123) = 534 – 531.2 = 2.8, p > 0.05. No statistically significant main effects were included in this model. No significant interaction was discovered with any predictor and time, which indicated no significant change over time related to student GPA.
Scholarly rigor A student scoring high in scholarly rigor tends to work hard to interpret and achieve a deeper understanding of complex or abstract material. Scholarly rigor scores did not change significantly over time. A parsimonious random slope model was reduced from the main effects only model which was not statistically different, χ 2 (5, N = 83) = 469.9 – 469.1 = 0.8, p > 0.05. The statistically significant main effect in this model was GPA in science. Students scoring higher in previous science courses tended to score higher than their peers. No significant interactions were discovered with any predictor and time, which indicated no significant change over time related to student GPA.
Conclusion
Mental focus changes over time were negatively correlated with science GPA, meaning the initial score differential (between higher and lower science GPA students) was decreased over time. This statistically significant reduction of the mental focus gap between higher and lower GPA students held a practical significance as mid and high GPA students showed a small decrease in mental focus, while low GPA students showed a more dramatic increase in focus over time. In contrast the mental focus gap between higher and lower mathematics GPA students was increased over time identified in the positive significant interaction between mathematics GPA and time.
Learning orientation and cognitive integrity were not significantly correlated with cumulative GPA or individual GPAs for math, science, or communications. Students began the semester with a score of approximately 32.4 and 34.2 (scale 0-50) in learning orientation and cognitive integrity, respectively. This indicated that students were “somewhat disposed” to desire an increase in their knowledge, skill base, truth seeking, and open-mindedness ( Insight Assessment, 2006 ). Small, but not statistically significant, increases over time were observed. No significant correlations were discovered with GPA or GPA interacting with time. This indicated that, regardless of GPA, students were equally likely to be interested in increasing knowledge and skill with a fair-minded perspective. A lack of correlation with GPA and time as an interaction factor indicated that higher achieving students did not change over time differently than their lower achieving counterparts.
Creative problem solving was slightly positively correlated with science GPA. Students with higher GPA in science tended to have a higher creative problem solving score, approximately 1.9 points (scale 0 to 50) higher per point on the GPA scale. Mean creative problem solving scores in October were 29.75, and statistically significant gains by April yielded a mean of 32.29. While two point gains held questionable practical significance, the average student did transition from “ambivalent” to “somewhat disposed” to having an increased tendency to approach problem solving with innovative or original ideas and solutions ( Insight Assessment, 2006 ). A slight negative correlation was observed with communications GPA, indicating that higher communication GPA students scored lower on creative problem solving. Gains over time were not correlated to any of the GPA data, which indicated that students, regardless of GPA, tended to increase creative problem solving scores over time at a similar rate.
Scholarly rigor was positively correlated with science GPA. Students with a higher GPA in science tended to score higher in scholarly rigor, approximately 2.1 points (scale 0 to 50) higher per point of GPA in science. Change over time was not statistically significant, nor was it correlated with GPA. Thus, student growth, over time, was unrelated to GPA in science, mathematics, or communications. Student mean scholarly rigor scores in October were 27.75 which increased, but not significantly, to 28.11 in April. This indicated that students were “ambivalent” in their disposition to work hard to interpret and achieve a deeper understanding of complex or abstract material ( Insight Assessment, 2006 ).
Supporting the existing literature base ( Dally & Zhang, 1993 ; Dunlap, 2005 ; Griffith, 2005 ; Lentz & Boe, 2004 ; Ricks, 2006 ; Rogers, 2005 ; Romero, et al., 2006 ; Roselli & Brophy, 2006 ; Weir, 2004 ), average motivation, measured pre and post did show improvement. In each of the five subscales of mental motivation, mean scores increased with the exception of mental focus which remained constant.
Discussion
As teachers introduce engineering design concepts into their classrooms, consistent with the Standards for Technological Literacy: Content for the Study of Technology ( International Technology Education Association, 2000 ), attention should be given to students’ motivation. Motivated students tend to be more easily engaged in learning activities and motivation is a desirable student characteristic to develop. Results of this study suggested that student motivation increased during the engineering design activities. However, mental focus is a subscale that presented a unique interaction with time and both science and mathematics GPA. Educators concerned with increasing motivation of students who are struggling may find these results encouraging. While engineering design activities rely on application of science principles, less academic students engaged in these experiences are showing increased focus over time. The Committee on K-12 Engineering Education may have offered an explanation of the results:
In theory, if students are taught science and mathematics concepts and skills while solving engineering or engineering-like problems, they will be able to grasp these concepts and learn these skills more easily and retain them better, because the engineering design approach can provide real-world context to what are otherwise very abstract concepts. ( Katehi, et al., 2009 , p. 51)
Data from this study were unable to explain why highly focused students (with higher science GPAs) showed losses over time. This discovery warrants further investigation but suggested that highly academic students were disengaging over time. It may be the case that instruction in this classroom catered to the middle and lower achieving students and was not developmentally appropriate for the advanced students thus, they were not adequately challenged.
The absence of a significant correlation between four of the five motivation subscales indicated that the introduction of engineering concepts did not have a differential impact on students of various academic backgrounds. This result preserved the positioning of technology education in a general education context. The finding of this study was that students struggling academically were not disadvantaged in terms of motivation as they encountered and experienced engineering design thinking processes. All students benefited from an education that included engineering design thinking.
Recommendations for Future Research
Students in this study were measured during one academic year. This single snapshot of a child’s development showed some growth in motivation and may represent a larger pattern. In 12 years of education and potentially post secondary education, does the growth pattern identified and discussed above continue? The holistic impact of a multiyear sequence of articulated technology courses may have some synergistic benefits for students beyond the simple sum of the parts. Educators and policy makers may be better able to allocate resources to support technology education with evidence that students exhibit a sustained increase in motivation.
Students who are highly successful in previous science courses demonstrated a reduction in metal focus while their less successful peers gained. This finding warrants further investigation. Why did these students decline in their motivation? One hypothesis is that the course was not substantially challenging for these students. An alternative hypothesis is that these students were successful in very structured programs of study and found the open ended application of scientific principles to be frustrating. The later hypothesis suggests another complex question about the differences between successful students in mathematics and science. Highly successful students in mathematics did not show a significant decline in motivation in this study.
In this study, students designed, fabricated, tested and redesigned electric powered vehicles. Further research might attempt to isolate what factors regarding design yield the greatest results in terms of motivation and its impact on student learning. Changing the Conversation ( National Academy of Engineering, 2008 ) suggested that the public conception of engineering be focused on the societal impacts of solutions. The design challenge for this study was related to developing alternative power technology for transportation. To what extent are the potential environmental and social impacts a factor in the student’s interest and motivation in this project? The educator’s choice of design challenge may impact student’s motivation related to the solution’s potential impact on society. Additional study might seek to identify the impact of limiting solutions to conceptual design rather than full implementation cycles. Can high school students learn (and be motivated to learn) successfully from conceptual design, or do they benefit substantially from implementation of the designed solution based on the experiential feedback of success and failures with opportunities for redesign and testing? Full implementation of student designs has the potential to engage the psychomotor domain of student development. Historically, technology education has been very successful and highly regarded for its ability to engage students’ psychomotor domains. Further study may discover a link facilitated through design implementation that successfully engages psychomotor, cognitive and affective domains simultaneously.
Acknowledgement
This material is based on work supported by the National Science Foundation under Grant Number ESI-0426421. Any opinions, findings, and conclusions or recommendations expressed in this material are those of the author(s) and do not necessarily reflect the views of the National Science Foundation.
Nathan Mentzer is an Assistant Professor at Purdue University. He can be reached at
nmentzer@purdue.edu.
Kurt Becker is a Professor and Department Head at Utah State University. He can be reached at
kurt.becker@usu.edu.
References
Bates, D., Maechler, M., & Dai, B. (2008). lme4: Linear mixed-effects models using S4 classes (Version 0.999375-22).
Bransford, J. D., Brown, A. L., & Cocking, R. R. (Eds.). (2000). How People Learn: Brain, Mind, Experience, and School: Expanded Edition Washington, D.C.: National Academy Press.
Dally, J. W., & Zhang, G. M. (1993). A Freshman Engineering Design Course. Journal of Engineering Education, 82 (2), 83-91.
Dunlap, J. C. (2005). Problem-Based Learning and Self-Efficacy: How a Capstone Course Prepares Students for a Profession. Educational Technology Research and Development, 53 (1).
Electrathon America (2007). Electrathon America Electric Vehicle Competition Retrieved July 15, 2007, from http://electrathonamerica.org/
Griffith, D. S. (2005). FIRST Robotics as a model for experiential problem-based learning: A comparison of student attitudes and interests in science, mathematics, engineering, and technology. Unpublished Dissertation, Clemson University.
Hox, J. (2002). Multilevel Analysis Techniques and Applications. New Jersey: Lawrence Erlbaum Associates.
Insight Assessment (2006). California Measure of Mental Motivation: Score Interpretation Document. Millbrae: Insight Assessment.
Insight Assessment (2007a). California Measure of Mental Motivation User Manual Retrieved July 15, 2007, from http://www.insightassessment.com/pdf_files/Manual%20CM3%202006.pdf
Insight Assessment (2007b). Tools to Evaluate Reasoning and Critical Thinking Retrieved July 15, 2007, from http://www.insightassessment.com/test-cm3.html
International Technology Education Association (1996). Technology for All Americans: A Rationale and Structure for the Study of Technology. Reston, VA: Author.
International Technology Education Association (2000). Standards for Technological Literacy: Content for the Study of Technology. Reston, VA: Author.
Katehi, L., Pearson, G., & Feder, M. (Eds.). (2009). Engineering in K-12 Education. Washington, D.C.: The National Academies Press.
Lentz, K., & Boe, N. (2004). Implementing Technology in Elementary Schools. Technology and Children, 9 (2), 19-20.
Lewis, T. (2004). A Turn to Engineering: The Continuing Struggle of Technology Education for Legitimization as a School Subject. Journal of Technology Education, 16 (1).
National Academy of Engineering (2008). Changing the Conversation: Messages for Improving Public Understanding of Engineering. Washington, D.C.: The National Academies Press.
Ricks, M. M. (2006). A study of the impact of an informal science education program on middle school students' science knowledge, science attitude, STEM high school and college course selections, and career decisions. Unpublished Dissertation, The University of Texas at Austin, Austin.
Rogers, G. E. (2005). Pre-engineering's Place in Technology Education and Its Effect on Technology Literacy as Perceived by Technology Education Teachers. Journal of Industrial Teacher Education, 42 (3), 6-22.
Romero, N. Y. d., Slater, P., & DeCristofano, C. (2006). Design Challenges are "ELL-ementary". Science and Children, 43 (4), 34-37.
Roselli, R. J., & Brophy, S. P. (2006). Effectiveness of Challenge-Based Instruction in Biomechanics. Journal of Engineering Education, 95 (4), 311-324.
U.S. Census Bureau (2000). Profile of General Demographic Characteristics: 2000 Retrieved May 27, 2009, from http://www.census.gov/prod/cen2000/dp1/2kh00.pdf
Weir, J. A. (2004). Active Learning in Transportation Engineering Education. Unpublished Dissertation, Worchester Polytechnic Institute.