JSTE v48n3 - Learning Effects of Design Strategies on High School Students
Learning Effects of Design Strategies on High School Students
John Mativo
Robert Wicklein
University Of Georgia
Abstract
An experimental design research method was used to study learning effects of design strategies comparing engineering design processes with trial and error design approaches. Students that met participation requirements were randomly selected and assigned into one of the two high school groups. The engineering design process was identified as the experimental treatment group while the trial and error process was identified as the control group. A common design project was created as the central focus for the instructional topic in both the experimental and control group for the study. Researchers collected end of treatment data from the student participants at the completion of a five-day program period. A one-way ANOVA was used to evaluate data from the Engineering Design Test . Analysis revealed an F -value of 4.398 with a significance of 0.043 between groups. A Cohen’s d effective size of -0.680 was realized, indicating a practical medium effect.
Learning Effects of Design Strategies on High School Students
Educators within technology education have had much debate over the benefits of engineering instruction within the field. Some educators advocate that engineering-based instruction can elevate the field of technology education to higher academic and technological levels and provide an ideal platform for integrating mathematics, science, and technology ( Wicklein, 2006 ). Individual technology teachers and some state departments of education have made attempts to develop engineering-based curricula. Additionally, nationalized curricula like Project Lead the Way and Engineering by Design support engineering-related instruction for the secondary level. However, it is evident from an examination of the literature that there are certain aspects inherent to the engineering design process that are not included in most of the engineering-based instruction developed by teachers and nationalized curricula ( Wright, 2002 ). Too often, engineering instruction taught within the technology education classroom is based on a trial and error approach to solving technological problems ( Hailey, Erekson, Becker, & Thomas, 2005 ). Although engineering-related terms may be used within this methodological structure, the central features of working within constraints and predictive analysis is either omitted completely or addressed in a cursory fashion.
There is little to no quantitative data that can be generalized to the field that pertains directly to the learning effects of predictive analysis as a basis for teaching engineering design. Predictive analysis requires the use of mathematical and scientific strategies to evaluate a potential design solution prior to ever creating a physical artifact or model. Engineers employ this process as a common practice to determine which potential design possibility would best solve a given technological problem. This research sought to add to the knowledge base related to utilizing an engineering design process which included predictive analysis within a high school technology education instructional format.
With respect to infusing engineering design into K-12 technology education, scholarly research has focused on student competencies in mathematics and science, instructional needs for teaching engineering design, student interest in STEM subjects, and pedagogical methods in integrating STEM subjects into an engineering framework ( McKenna and Agogino, 1998 ; DeGrazia, Sullivan, Carlson, & Carlson 2001 ; Dearing and Daugherty, 2004 ; Richards and Schnittka, 2006 ; Ross, Bayles, & Titus 2006 ; Spence, Bayles, & Corkum 2006 ). In a recent study of 283 in-service technology teachers, Gattie & Wicklein (2007) found that most technology teachers (90 percent) considered themselves to be teaching courses and topics related to engineering or engineering design and that almost half of their instructional time (45 percent) was committed to this activity. The majority of technology teachers surveyed in Gattie and Wicklein’s research viewed engineering design as the appropriate focus for technology education. Cunningham, Knight, Carlsen, & Kelly, (2007) suggested that teachers’ lack of knowledge about engineering was one of the principle obstacles that must be overcome in order to successfully integrate engineering concepts into middle and high school classrooms. This view was further expressed by a study within the National Academy of Engineering ( NAE, 2009 ), which placed the deficiency at the feet of teacher preparation programs, stating “One significant deficiency we observed in engineering professional development at the secondary level is a lack of critical analysis and reflection on pedagogy per se” (p. 23). In a study to identify appropriate learning outcomes for high school technology education students with an engineering design focus, Rhodes and Childress (2006) identified the number one necessary outcome as the ability to identify problems that could be solved through engineering design. Clearly, understanding what constitutes an appropriate and effective engineering design problem for secondary level students is essential in order to teach engineering design authentically and accurately. In addition to identifying appropriate design problems for the classroom, the ability to teach appropriate engineering design strategies is another crucial element. Currently most engineering-based instruction at the high school level is based on a trial and error approach to solving technological problems. This approach employs the process of students randomly selecting solution strategies that are based on hunches rather than mathematical and/or scientific applications. Using trial and error overlooks an essential feature of the engineering design process, which is the use of analytical predictive analysis to determine best options for solving a problem. Without a clear and distinct application of predictive analysis, any technological problem-solving effort would be severally limited in its results and would not be an effective method of solving most real-world problems. Unfortunately, predictive analysis is often omitted or only moderately addressed in many technology education programs. The effect of the many technology education programs that utilized a trial and error approach in solving technological problems was that students did not have an opportunity to engage in mathematical and scientific applications. Students are often left with the understanding that engineering is no more than a series of basic trial and error approaches to technological problem solving, which is incorrect and misleading.
Further, real-world technological or engineering design problems are usually ill-defined, complex, and vague ( Dym, Agogino, Eris, Frey, & Lieifer, 2005 ). Based on the Accrediting Board for Engineering and Technology (ABET) standards, engineering design is the process of devising a system, component, or process to meet desired needs. It is a decision-making process (often iterative and replicating), in which the basic sciences, mathematics, and engineering sciences are applied to convert resources optimally to meet stated needs (ABET, 2007). To successfully solve engineering design problems, designers undergo the process of identifying the needs and constraints connected with the problem, generating solution ideas, and evaluating the solutions that will satisfy the users’ and customers’ needs ( Dym, 1994 ). Khandani (2005) suggested the engineering design process should include defining the problem, gathering pertinent information, generating multiple solutions, analyzing and selecting a solution, and testing and implementing the solution.
During the last two decades, the complex, uncertain, and dynamic nature of real-world problem solving has interested cognitive psychologists (e.g., Sinnott, 1989 ; Voss, Wolfe, Lawrence, & Engle, 1991 ), applied psychologists (e.g., Zsambok & Klein, 1997 ), educational psychologists (e.g., Spiro, Feltovich, & Coulson, 1996 ), and instructional technologists (e.g., Jonassen, 1997 ; Shin, Jonassen, & McGee, 2003 ). This stream of research focuses on the differences in the characteristics of well-defined problems, often used in classrooms ( Spiro, Feltovich, Jacobson, & Coulson, 1992 ), and ill-defined problems, most common in the real world. One of the essential findings from previous studies was that well-structured and ill-structured problem-solving activities required different kinds of skills and abilities (e.g., Schraw, Dunkle, & Bendixen, 1995 ). In fact, many problems encountered every day pose uncertainties in various ways, including the complexity of the problem context; multiple and often conflicting perspectives among stakeholders; diverse solutions or no solution; and multiple criteria for solution evaluation. These are the general features of ill-defined problems ( Jonassen, 1997 , 2000 ; Kitchener, 1983 ; Shin et al., 2003 ; Wood, 1983 ). The complexity of the problems, the existence of conflicting perspectives, and the potential for multiple solutions do not merely make the problems more sophisticated; rather, these features change the nature of the problems. Thus, ill-defined problem solving demands a different set of intellectual skills and attitudes that may not be necessary conditions for solving well-defined problems that have clear goals and known rules to apply ( Jonassen, 1997 ; Schraw et al., 1995 ; Shin et al., 2003 ). All too often secondary level students within technology educations programs in the United States are limited or are completely void of exposure to ill-defined problem solving.
Theoretical Foundation
The theoretical or conceptual framework for this study was based on the general models of design that were formulated and established by the International Technology Education Association (ITEA) and various educators within the field of engineering education ( Burghardt, 1999 ; Eide, Jenison, Mashaw, & Northup, 2002 , 2008 ; Koen, 2003 ). Table 1 expresses the similar yet unique differences between what these two design models are describing as (a) Technology Education Design and (b) Engineering Design processes. The two design scenarios provided the basis for the theoretical framework for this research study. The highlighted text in italics represent the unique differences that guided the focus of this study ( bold italic = Engineering Design Process and italic = Technology Education Design Process).
Table 1: Comparison of Engineering Design and Technology Education Design Process
Engineering Design Process
(Eide, Jenison, Mashaw, & Northup, 2002)
- Identify the Need
- Define the Problem
- Search for Solutions
- Identify Constraints
- Specify Evaluation Criteria
- Generate Alternative Solutions
- Analysis
- Mathematical Predictions
- Optimization
- Decision
- Design Specifications
- Communication
Technology Education Design Process
(Standards for Technological Literacy, 2000)
- Defining a Problem
- Brainstorming
- Researching & Generating Ideas
- Identifying Criteria
- Specifying Constraints
- Exploring Possibilities
- Select an Approach and Develop a Design Proposal
- Building a Model or Prototype
- Testing & evaluating the Design
- Refining the Design
- Communicating Results
Method
The research question and protocol for this project are explained in this section as well as explanations of the primary research activities that were accomplished during the project. A null hypothesis was established to guide this study and is stated as:
There is no significant statistical difference in engineering design learning ability for students who participated in an engineering activity based on predictive analysis when compared with an engineering activity based on trial and error.
Identification of School Partner. The target population for this research came from a high school located in Northeast Georgia. High school students at the 11 th and 12 th grade level were the potential participants for this experimental research.
Selection of Participants. A total of 40 high school students (11 th and 12 th graders) were randomly selected for this project. During April of 2009, a letter was sent to all qualified 11 th and 12 th grade students inviting them to participate in a special program during the summer of 2009. Qualification of students to be selected for this experiment was based on academic standing (GPA 2.5 or higher), in good standing with the selected high school, and parent/guardian permission to participate in the study. Based on the responses from the potential participants, 40 students were randomly selected to participate in the study.
Random Assignment of Participants. From the total pool of participants, the students were randomly assigned to either the experimental treatment group (predictive analysis group) or the control group (trial and error group). Each group had a total of 20 participants. Within each group, students were further randomly assigned to one of five (5) development groups with four (4) students per group. This was done to allow each member of the group to take part in the solution process.
Selection of Instructional Topic and Preparation for Instructor Training. As presented at the end of this paper, a soft drink (aluminum) can crusher design challenge was selected for the instructional content of this experiment. This instructional activity was selected based on its perceived appropriateness as an engineering-related technological problem and was deemed age and gender suitable by the researchers. The activity involved the design and development of a soft drink (aluminum) can crusher device that would require students to use their knowledge about lever systems and calculations that result in mechanical advantage. Researchers viewed this as a classic problem that could be solved in an analytical manner, based on the level of student knowledge related to physics lever mechanisms and mathematical concepts. The challenge was deemed to be gender free, because anyone could own and operate a soft drink can crusher without discrimination. The instructional activity content was designed to be completed in five days with three-hour per day class sessions. Assumptions made to determine activity completion rates were based on predictive analysis and trial and error processes taking place within the first day. The second, third, and fourth days were to be spent in the laboratory implementing day one decisions and tweaking those decisions as needed. Presentation and evaluation day was the fifth day, where students would explain and demonstrate their process to problem solving.
Selection and Training of Instructors. A purposeful selection of two technology education teachers to participate in this study was done from a local pool. Each teacher was a veteran of the classroom (five or more years of teaching experience at the high school level) and had knowledge of the design process. The teacher whose school hosted the experiment was assigned the treatment group while the second teacher was in charge of the control group. They underwent training in the objectives of the research study. After this assignment, each underwent further training regarding the instructional topic and the instructional methodology that each would use during the program of instruction (experimental group = predictive analysis and control group=trial and error). The experimental group teacher received training in classical lever class problems and mechanical advantages that accompany such arrangements. The control teacher received the traditional technology education approach to solving a problem through a trial and error method. Teachers were supplied with the appropriate laboratory and written materials needed for the instructional programs. Instructors were required to follow a strict instructional regiment that aligned with their assigned instructional methodology.
Preparation of Classroom and Laboratory Facilities. The research was conducted at a high school in northern Georgia within the technology education classroom facility of that school. The facility was divided in two, with one section populated with more than twenty computers for students to use as needed, and the second section a laboratory with hand tools and machines that would support the instructional activity solution. The arrangements of the classroom/laboratory facility were identical for both the experimental and control groups. Students could move in and out of the two rooms as needed. Instructional materials were prepared by the research staff and supplied to the teachers.
Conducting the Instructional Programs. The program was implemented during a five-day period in mid-June 2009. Instruction for the experimental group took place from 9:00 a.m. to 12:00 p.m., and instruction for the control group took place from 1:00 p.m. to 4:00 p.m. The selection of the two different times was done to prevent cross-talk among the student participants. At the completion of the instructional period, all students were administered a standardized Engineering Design Test that measured capability to understand and apply a comprehensive engineering design process. The Engineering Design Test had been tested and revised for validity and reliability.
Data Collection and Analysis. Data was collected from the student participants at the completion of the five-day program period. All data was quantitative and based on student responses to the Engineering Design Test. Once data had been collected, it was entered into the SPSS statistical software. An analysis of variance (ANOVA) was conducted to determine differences between groups and within groups. Further, to investigate whether a practical effect existed, a Cohen’s d analysis was performed. These analysis methods provided the statistical results to enable the comparison of the design methods.
Results and Discussion
Analysis addressing the research question, Is there a significant difference in engineering design learning ability for students who participated in an engineering activity based on predictive analysis when compared with an engineering activity based on trial and error? , yielded an F value of 4.398 having significance at 0.043 for between the groups, indicating that there is a statistical significant difference in engineering design learning ability between the two student groups. Table 2 indicates the results of the ANOVA.
Sum of squares | df | Mean Squares | F | Sig. | |
---|---|---|---|---|---|
Between Groups | 70.225 | 1 | 70.225 | 4.398 | 0.043 |
Within Groups | 606.750 | 38 | 15.967 | ||
Total | 676.975 | 39 |
To further investigate whether a practical effect existed, a Cohen’s d analysis was used to determine the effect size for the difference between groups that range from 0.2 (small effect), 0.5 (medium effect), and 0.8 (large effect) (Cohen, 1988). For this group, a Cohen’s d of -0.68 resulted. This result indicated that a practical effect existed between the two student groups. From a possible score of 36 on the Engineering Design Test , the treatment group (predictive analysis group) had a mean of 20.65 (SD=4.49) on the test, while the control group (trial and error group) had a mean of 23.30 (SD=3.44). The mean scores indicated that the trial and error group performed better than the treatment group.
The researchers sought to understand the reasons behind the differences in the group test scores, especially since the null hypothesis was rejected in the opposite direction of the expected results. The following explanations provide the researchers thoughts and conclusions to explain why the results of the experiment yielded this effect.
- Although the instructional activity was well thought through and considered with regard to age, gender, and topical relevance the issues related to identifying appropriate instructional topics that lend themselves to incorporating predictive analysis strategies that are in-line with student prior knowledge of mathematics and science is difficult to determine. The researchers were not aware of any past educational research which identified instructional topic selection with regard to the incorporation of predictive analysis strategies for secondary level students. Further research needs to be conducted to help identify the critical and essential features that should be included in instructional activities that seek to help high school students engage in engineering design processes that include the components of predictive analysis.
- Time that was devoted to student’s learning the engineering design process was limited and was determined to be too short. The 12 hours of instructional time within this experiment was insufficient for students in the treatment group to gain a full understanding and appreciation of the predictive analysis procedures that were needed to solve the instructional activity. Inversely, the control group did not need significant additional learning time to employ the trial and error strategies that students used to solve the instructional activity since this was the common default approach to solving technological problems. Tying together mathematics and science concepts and relating them to physical solutions to technological problems must be orchestrated in a fully articulated way that is both systematic and repetitive in order to build confidence and effectiveness by the student user. In this research, the treatment group was exposed to both physics and mathematics that pertain to lever systems. The reason for the exposure was to help students relate to the lever classes and apply their basics to the project design. Few student teams within the group attempted to use this knowledge in their design and abandoned most forms of this knowledge due to pressure of time and limited confidence in the use of the computational manipulation that would be translated into physical reality as a solution. Could it be that the treatment group did not reach their goal due to circumstantial factors rather than the learning strategy that was intended? This question is worth investigating in future experiments. A prolonged amount of instructional time on relevant learning activities culminating with an appropriate learning challenge should be considered when teaching engineering design to high school students.
- The Engineering Design Test was not sensitive enough to measure the subtleties between the two student groups. The Engineering Design Test was created to measure student knowledge of the engineering design process and may not be able to discriminate the unique variations of student prior experiences in technological problems solving. A further examinations and refinement of the Engineering Design Test is currently underway.
- Small population size. Limitations of the small numbers in each group may have been a factor in the differences between treatment groups. Further research needs to be done with larger numbers of students where random selection and assignment can be accomplished with samples from more complete populations.
- Time of day effects could have caused a difference. Although random selection and random placement of the participants was conducted, there could be unknown elements that worked in favor of the control group that met in the afternoon over the treatment group that met in the morning. Considering that the study took place in the summer and 11 th and 12 th graders stay up late during summer – although there is no data to support the assertion nor do the researchers believe this to be a factor – they suggest that an element of not being fully awake for the morning group (predictive analysis group) be investigated for any potential contribution to the outcome. Future research is recommended for the time of day to minimize the day effect.
The reasons observed above raise practical and critical questions in determining how much time, curriculum design, and instructional methodology is required to adequately integrate a new approach to build engineering design content knowledge in high school students. The time required to build and integrate the essential instructional and learning tools that will yield students connecting and applying STEM (science, technology, engineering, mathematics) content is still unknown. Incorporating the engineering design process that includes significant uses of the predictive analysis process seems to be a logical approach to connecting STEM content together; however, much more research will need to take place before educators can be successful with this approach.
Initial learning of content, whether science, technology, engineering, or mathematics, is necessary for transfer to other areas and topics. The National Research Council ( NRC, 1999 ) discussed how learning involves transfer on previous learning. This explanation of learning transfer identified three variables crucial to learning: (1) degree of mastery of the original work, (2) degree to which people learn with understanding rather than merely memorized sets of facts or following a fixed set of procedures, and (3) the amount of time it takes to learn material is proportional to the material being learned. Further, the discussion suggested that attempts to cover too many topics too quickly could hinder learning and subsequent transfer because students would learn isolated sets of facts that may not be organized and connected, or they are introduced to organizing principles that they cannot grasp because they lack enough specific knowledge to make them meaningful. These are factors that likely contributed to the outcome of the study in classic engineering programs; students pull from different resources of courses taken to create a solution for a given problem. This approach provides ample time to build up the intellectual capacity and affective characteristics that enable one to make an analytical approach realistic.
Conclusion
To strengthen and better understand the impact of future experiments, the researchers suggest the inclusion of measurements of mathematics and science gains from the experiment activity. Measured gain is the value added component to student understanding and use of new concepts, skills, and/or attitudes that have been acquired through participation in a learning event. Future experiment designs are encouraged to establish student current knowledge level and expected knowledge level after participation. This measurement could be achieved by administering a pre-assessment survey to the students at the beginning of the experiment and a post-assessment survey at the end of the activity and comparing outcomes of both assessments. The difference in post and pre assessment is the value added. The value added will inform whether participation in the experiment design contributes towards the learning of new material and help develop intended higher level of knowledge transfer. The higher level of knowledge transfer would equip the participant with a generalized approach to problem solving activities that shapes individual methods of design to solve various problems with effective solutions.
This study was set to investigate learning effects of design strategies using an engineering design process versus a trial and error approach. The outcome revealed that the control group (trial and error) performed significantly better than the treatment group (predictive analysis group). The researchers sought reasons that could have led to this outcome and observed that, time constraints in integration of predictive analysis tools was the likely factor that led to lower performance by the treatment group. The selection and design of the instructional activity used in the experiment may have also contributed to the results of this study. Hatamura (2009) advised that the best approach in human activity is to act wisely, acquire knowledge of potential failures and let knowledge guide actions.
The researchers propose that adequate preparation be given to all those that use the engineering design process as a means to teach engineering content and engage in STEM education. This preparation should include immersion in both mathematics and scientific concepts and more importantly, rigorous applications in several problems with known results before students attempt to solve ill-defined problems that require significant levels of learning transfer.
Author Note
This research was supported by NCETE Award #029485-08 grant. Correspondence concerning this paper should be addressed to Dr. John M. Mativo, Workforce Education/Faculty of Engineering, 213 River’s Crossing Building, 850 College Station Road, Athens, GA 30602-4808. Email: jmativo@uga.edu , Phone: (706) 583-8107
Author
John Mativo is an Assistant Professor at the University of Georgia. He can be reached at jmativo@uga.edu .
Robert Wicklein is a Professor at the University of Georgia. He can be reached at wickone@uga.edu .
References
Burghardt, M. D. (1999). Introduction to Engineering Design and Problem Solving. McGraw Hill, Inc.
Cohen, J. (1988). Statistical power analysis for the behavioral sciences (2 nd Ed.). Hillsdale, NJ: Lawrence Earlbaum Associates
Cunningham, C. E., Knight, M. T., Carlsen, W. S., & Kelly, G. (2007). Integrating engineering in middle and high school classrooms. International Journal of Engineering Education , 23(1) 3-8.
Dearing B. M. & Daugherty, M. K. (2004). Delivering engineering content in technology education. The Technology Teacher, 64(3): 8-11.
DeGrazia J.L., Sullivan J.F., Carlson L..E, Carlson D.W. (2001). A K-12/university partnership: creating tomorrow’s engineers. Journal of Engineering Education, 90(4):557-563.
Dym, C. L. (1994). Engineering Design: A Synthesis View. Cambridge: Cambridge University Press.
Dym, C. L., Agogino, A. M., Eris, O., Frey, D. D., & Leifer, L. J. (2005). Engineering design thinking, teaching, and learning. Journal of Engineering Education, 94 (1), 103 – 120.
Eide, A. R., Jenison, R.D., Mashaw, L.H., Northup, L.L. (2002). Introduction to Engineering Design and Problem Solving. (2 nd ed.). Boston, McGraw Hill, Inc.
Eide, A. R., Jenison, R.D., Mashaw, L.H., Northup, L.L. (2008). Introduction to Engineering Design and Problem Solving. (5 th ed.). Boston, McGraw Hill, Inc.
Gattie D.K. & Wicklein, R. C. (2007). Curricular value and instructional needs for infusing engineering design into K-12 technology education. Journal of Technology Education, Accepted for publication.
Hailey, C.E., Erekson, T., Becker, K., & Thomas, T. (2005). National center for engineering and technology education. The Technology Teacher. 64(5) pgs. 23-26.
Hatamura, Y. (2009). Learning from Design Failures. Hatamura Institute for Advancement of Technology. Springer, Tokyo, Japan
International Technology Education Association. (2000). Standards for Technological Literacy: Content for the Study of Technology. Reston, VA: International Technology Education Association.
Jonassen, D. H. (1997). Instructional design models for well-structured and ill-structured problem solving learning outcomes. Educational Technology Research and Development, 45(1), 65-94.
Jonassen, D. H. (2000). Toward a design theory of problem solving. Educational Technology Research and Development, 48(4), 63-85.
Khandani, S. (2005). Engineering design process: Education transfer plan. http://www.iisme.org/etp/HSEngineering-Engineering.pdf Accessed 11/11/2010.
Kitchener, K. S. (1983). Cognition, metacognition, and epistemic cognition. Human Development, 26 , 222-232.
Koen, B. V. (2003). Discussion of the Method: Conducting the Engineer’s Approach to Problem Solving. New York: Oxford University Press.
McKenna A. & Agogino, A. (1998). A web-based instructional module for teaching middle school students engineering design with simple machines. Journal of Engineering Education, 87(4):437-444.
National Academy of Engineering (2009). Professional development for teachers of engineering: Research and related activities. Custer, R. L. & Daugherty. The Bridge: Linking Engineering and Society. Vol 39 No. 3 Fall.
National Research Council (1999). How people learn: Brain, mind, experience and school. Commission on Behavioral and Social Science and Education.” (Bransford, J. D., Brown, A. L., and Cocking, R. R. Eds.) National Academy Press, Washington, D. C.
Rhodes, C., & Childress, V. (2006). Engineering outcomes for grades 9-12. Unpublished manuscript, The National Center for Engineering and Technology Education, North Carolina A&T, Greensboro.
Richards L.G. & Schnittka C.G. (2006). Bringing engineering into middle schools: Learning science and math through guided inquiry and engineering design. 2006 ASEE Workshop on K-12 Engineering Education.
Ross, J., Bayles, T., & Titus, S. (2006). INSPIRES (Increasing Student Participation, Interest and Recruitment in Engineering and Science). 2006 ASEE Workshop on K-12 Engineering Education.
Rogers, G.E. (2005). Pre-engineering’s place in technology education and its effect on technological literacy as perceived by technology education teachers. Journal of Industrial Technology Teacher, 42, (1) , 6-22.
Schraw, G., Dunkle, M. E., & Bendixen, L. D. (1995). Cognitive processes in well-defined and ill-defined problem solving. Applied Cognitive Psychology, 9, 1-16.
Shin, N., Jonassen, D. H., & McGee, S. (2003). Predictors of well-structured and ill-structured problem solving in an astronomy simulation. Journal of Research in Science Teaching, 40(1), 7-27.
Sinnott, J. D. (1989). A model for solution of ill-structured problems: Implications for everyday and abstract problem solving. In J. D. Sinnott (Ed.), Everyday Problem Solving: Theory and Applications (pp. 72-99). New York: Praeger.
Spence, A., Bayles, T., & Corkum, M., (2006). Introduction to engineering through mathematics. 2006 ASEE Workshop on K-12 Engineering Education.
Spiro, R. J., Feltovich, P. J., Jacobson, M. J., & Coulson, R. L. (1992). Cognitive flexibility, constructivism, and hypertext: Random access instruction for advanced knowledge acquisition in ill-structured domains. In T. M. Duffy & D. H. Jonassen (Eds.), Constructivism and the Technology of Instruction: A Conversation (pp. 57-75). Hillsdale, NJ: Lawrence Erlbaum Associates.
Spiro, R. J., Feltovich, P. J., & Coulson, R. L. (1996). Two epistemic world-views: Prefigurative schemas and learning in complex domains. Applied Cognitive Psychology, 10, s51-s61.
Voss, J. F., Wolfe, C. R., Lawrence, J. A., & Engle, R. A. (1991). From representation to decision: An analysis of problem solving in international relations. In R. J. Sternberg & P. A. Frensh (Eds.), Complex Problem Solving: Principles and Mechanisms (pp. 119-157). Hilldale, N. J.: Lawrence Erlbaum.
Wicklein, R. C. (2006). Five good reasons for engineering design as the focus for technology education. The Technology Teacher, 65 (7), 25-29.
Wood, P. K. (1983). Inquiry systems and problem structures: Implications for cognitive developments. Human Development, 26, 249-265.
Wright, P. (2002) Introduction to Engineering. John Wiley & Sons, Inc. Hoboken, NJ.
Zsambok, C. E., & Klein, G. (Eds.). (1997). Naturalistic Decision Making. Mahwah, NJ: Lawrence Erlbaum Associates.
Appendix
Soda Can Crusher Challenge
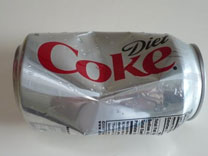
You may be an environmentally conscious person. You have noticed that your family buys and drinks significant amounts of soda. Your family may prefer to buy soda in regular 12 oz. or 355 ml aluminum cans. Here are some interesting facts about aluminum: Huge earthmover vehicles extract bauxite from the earth. The bauxite is then mechanically crushed to separate it from impurities before being transported to a smelting plant where high energy is used to melt and extract aluminum. The aluminum is then sent to factories for stamping and extruding to create the can that soda is placed in. Assuming the energy spent in this manner to develop a soda can is 100 percent, recycling would use only 5 percent energy to develop the same can. Recycling aluminum cans makes a lot of sense because it saves valuable energy.
YOUR CHALLENGE:
To help improve the process for recycling aluminum soda cans you and your team are to design, construct, and test a wall-mounted soda can crusher that will reduce a standard size and shape a 12 fl. oz. soda can to one inch in height. This reduction in height will aid in storing more cans in recycling bins and collection sites.
Design Specifications:
Design and product must address the following:
- Must be safe to operate
- Must be able to be operated with 2 lbs. of force
- Should be aesthetically pleasing
- Should be functional (reduce 12 fl. oz. aluminum can to 1 inch in height)
- Should be reliable (be able to crush 20 cans in 2 minutes)
- Must fit within the following dimensions: 24” (height) X 6” (width) X 6” (depth)
Constraints/Limitations:
- Produced from teacher supplied materials
- Produced using available laboratory tools
- Produced within the allotted time limit
Evaluation of Assignment:
- Detailed documentation of can crusher design
- Working can crusher product
- Functional test of can crusher
Evaluation Criteria Value | Points |
---|---|
Size Limitation of Product (24” X 6” X 6”) | 5 |
Safe Operation of Product | 10 |
Reliability/Durability of Product (10 can in 2 minutes) | 15 |
Detailed Documentation of Design Process | 20 |
Operation - Force Applied (5 lbs. force) | 25 |
Functional Product (can reduced to 1 ½” height) | 25 |
TOTAL | 100 |
Material | Size | Quantity |
---|---|---|
Wood Screws | 1 ½” | 20 |
Wood Screws | 1” | 20 |
Board | 2” X 4” X 45” | 1 |
Board | 1” X 6” X 3’ | 1 |
Plywood | ¼” X 2’ X 4’ | 1 |
Dowel Rod | 3/8” X 4’ | 1 |
Wood Glue | Capacity | Capacity |
Thumb Tacks | Normal | 5 |
Rubber Bands | ¼” X 2” | 4 |
Tool Used:
Tools used to construct the product must be done under the direct supervision of the instructor or research staff.
Evaluation Rubric
Evaluation Topic | Below Standard | At Standard | Above Standard | Specific Comments |
---|---|---|---|---|
Size Limitation of Product
(24” X 6” X 6”) 5 pts. Maximum |
Product not completed | Product constructed within size limitations | Efficient utilization of materials to construct product | |
Safe Operation of Product
10 pts. Maximum |
Product functioned in an unsafe mode | Product functioned safely | Product functioned safely and efficiently | |
Reliability/Durability of Product
(10 cans in 2 minutes) 15 pts. Maximum |
Product not able to perform required amount of processing within time period | Product able to process required amount of processing within time period | Product able to process required amount of processing prior to time period ending | |
Detailed Documentation of Design Process
20 pts. Maximum |
Use of engineering design notebook was inadequate and not complete | Use of engineering design notebook was adequate and complete | Use of engineering design notebook indicated superior understanding of documentation process | |
Operation – Force Applied = 5 lbs.
25 pts. Maximum |
Force needed to operate product exceeded 5 lbs. of force | Force needed to operate product was within operating force parameters (5 lbs. + 0.25 lbs. force) | Force needed to operate product was less than 5 lbs. force | |
Functional Product (Can crushed to 1 ½ inch height)
25 pts. Maximum |
Crushed can exceeded the 1 ½ inch height criteria | Crushed can was within the 1 ½ inch height criteria (1 ½ inch + 0.25 inches) | Crushed can was less than the 1 ½ inch height criteria |
This study was conducted to compare and evaluate the differences in student learning effects of engineering design taught from a trial error based approach versus a predictive analysis approach using an experimental design research method.